Integrated Multi-Omic Analysis:
Enhancing Mechanistic Insights and Biomarker Discovery
Panome Bio offers integrated multi-omic analysis through a proprietary bioinformatics pipeline that
interweaves ‘omics profiles into a singular dataset for higher-level analysis.
Enhance Mechanistic Insights
Integrated multi-omics provides enhanced mechanistic insight through broader and deeper biochemical pathway coverage.
Magnify Biomarker Discovery
An integrated multi-omic approach enhances biomarker discovery through the evaluation of more molecules in one sample, improving group separation and imparting confidence when observations from multiple analyte types co-cluster in one pathway or network.
Maximize Potential
The potential within multi-omic approaches is not realized by simply producing multiple ‘omic datasets, but by integrating the information provided with data-driven and literature-guided approaches. Integrated multi-omics data enables more meaningful pathway analysis, greater hit confidence, and reaction-level insights.
Improve Understanding of Disease
Disease states originate within different molecular layers (gene-level, transcript-level, protein-level, metabolite-level). By measuring multiple analyte types in a pathway, biological dysregulation can be pinpointed to single reactions, enabling elucidation of actionable targets.
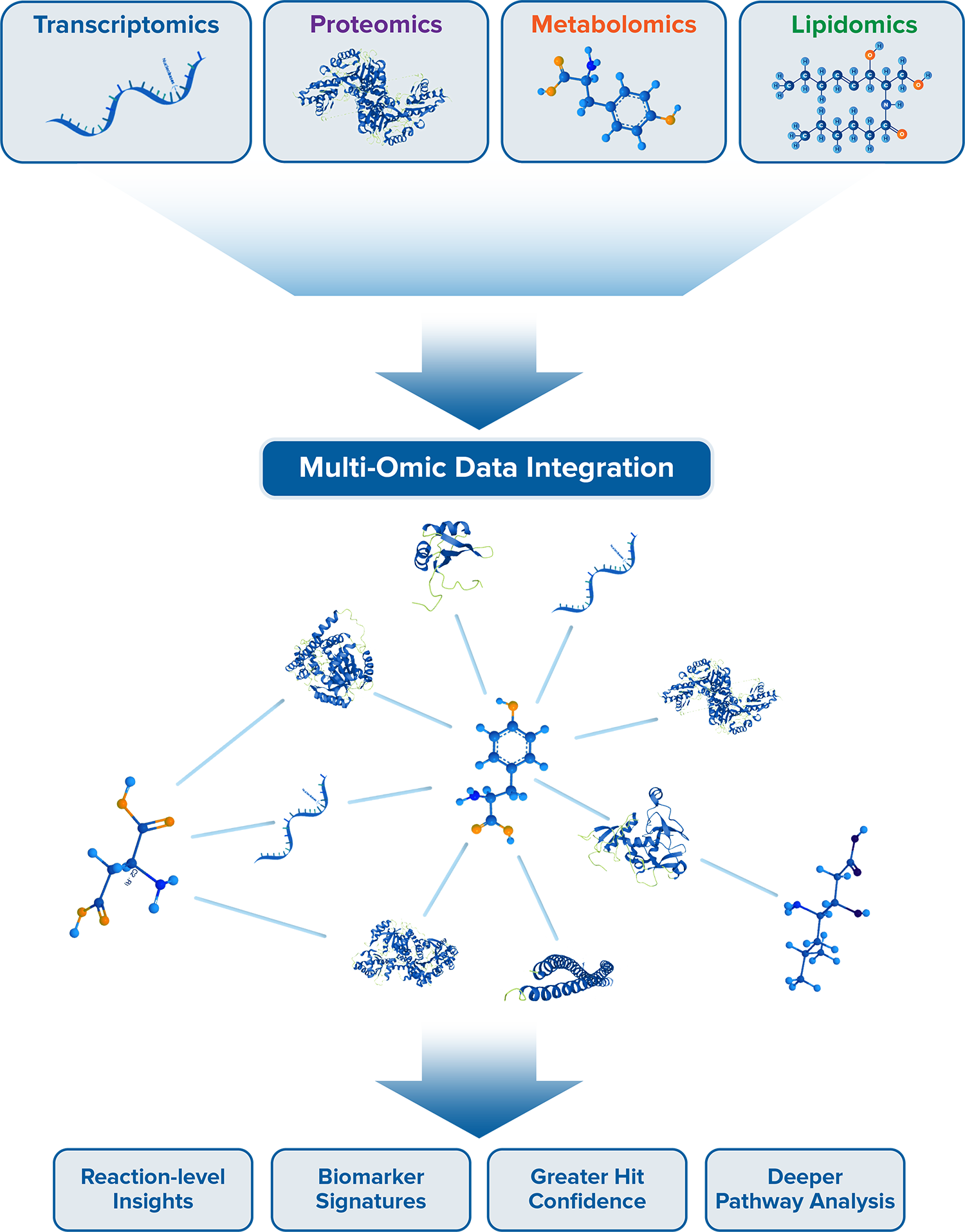